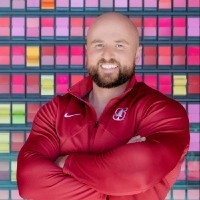
Low-performing teams saw a ~15% increase in productivity from the use of Generative AI tools, while high-performing teams saw just a ~6% increase.
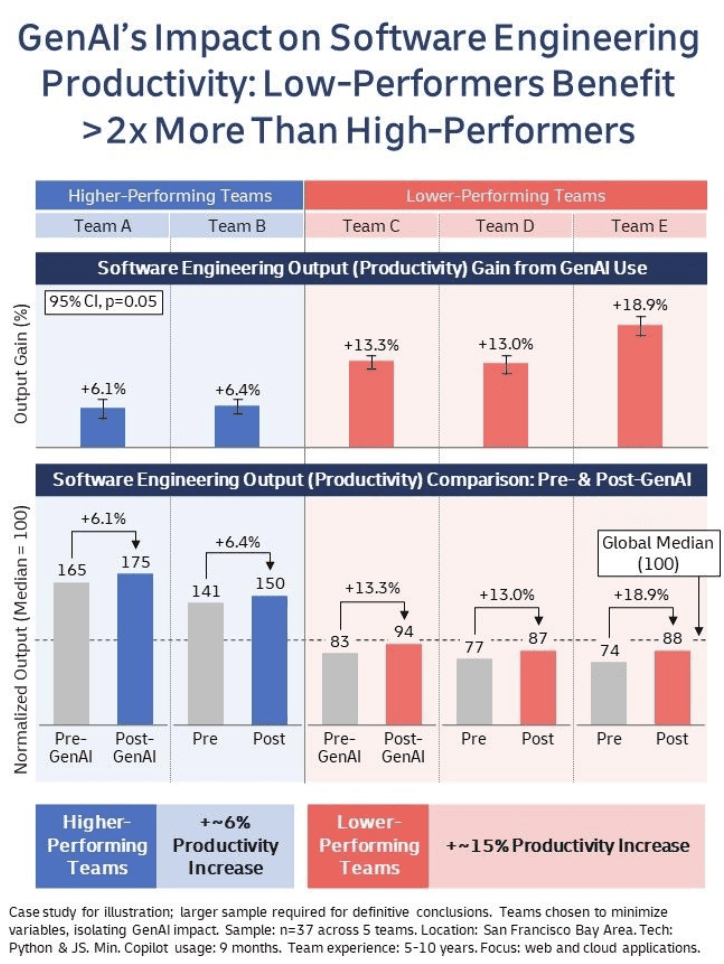
What could be the reasons behind this disparity?
In this case study, we examined 5 software teams using GenAI for >9 months. These teams were comparable in size, composition, location, programming language use, experience, turnover, GenAI tool use, and project type, allowing us to isolate the impact of GenAI.
We categorized them into high-performing (top ~30%) and low-performing (bottom ~30%) groups, analyzing their productivity before and after using GenAI for 9+ months each.
Despite its small sample size, this case study is a first in objectively evaluating GenAI's impact on software development productivity.
Traditional productivity metrics (Lines of Code, Commits/PRs, Story Points, DORA metrics) can misrepresent developer productivity: they don’t tightly correlate with productivity because they don’t analyze source code, might encourage counterproductive behavior, and could be easily manipulated.
How do we measure developer productivity in this Stanford case study?
Our algorithm measures developer productivity by analyzing the functionality (ie. what the code does) of code changes in Git commits. It weighs 30+ codebase dimensions and has been calibrated across millions of files in 10+ languages. This quantifies each commit's impact and allows us to combine it with Git metadata to measure individual and team productivity.
About Our Mission
-We are conducting research at Stanford focused on quantifying software engineering productivity.
-Our goal is to help engineering teams make decisions grounded in hard data, moving away from intuition and office politics.
-Participants in our research use our algorithm to make data-driven decisions about team performance, headcount, outsourcing, work settings (home vs office), etc.